· 9 min read
Unveiling the Power of Retrieval Augmented Generation
Explore how Retrieval-Augmented Generation (RAG) is enhancing AI model accuracy, real-time data integration, and contextual relevance across industries, particularly in enterprise solutions.
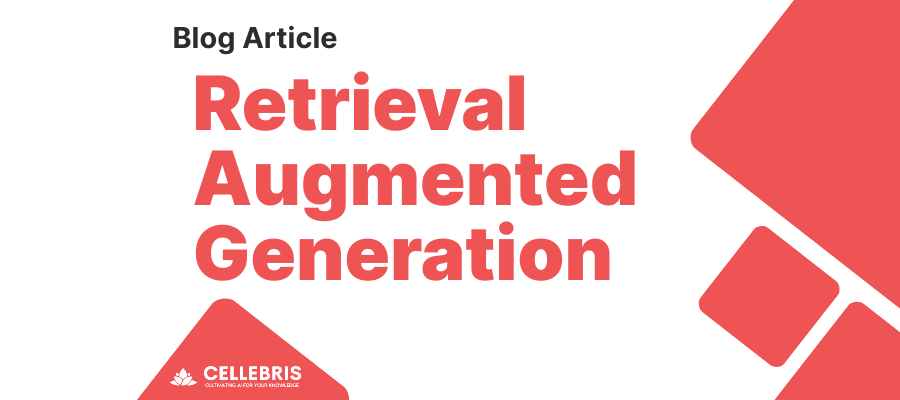
Introduction to Retrieval-Augmented Generation for Enterprises
In today’s rapidly evolving tech landscape, Retrieval-Augmented Generation (RAG) is emerging as a transformative technology that is redefining the capabilities of artificial intelligence (AI), particularly within enterprise applications. As businesses increasingly rely on AI to drive innovation, efficiency, and competitive advantage, the limitations of traditional AI models become more apparent. Conventional AI systems, often confined to pre-trained data sets, struggle to keep pace with the dynamic nature of real-world information. This is where RAG steps in, seamlessly blending the prowess of large language models (LLMs) with real-time data retrieval to deliver responses that are not only accurate but also contextually relevant and up-to-date.
By harnessing the capabilities of RAG, enterprises can overcome the challenges of static knowledge bases, ensuring that their AI-driven solutions are always aligned with the latest information. This approach not only enhances knowledge management and operational continuity but also equips organizations with the tools needed to address complex, real-world challenges effectively. Whether it’s providing customers with the most recent product information, aiding healthcare professionals with the latest medical research, or optimizing manufacturing processes with real-time data, RAG is poised to become an indispensable asset in the enterprise toolkit.
Key Topics Covered
- What is Retrieval-Augmented Generation (RAG)? Understanding the fundamentals of RAG and how it differs from traditional AI models.
- How RAG Boosts AI Performance and Scalability Exploring the mechanisms through which RAG enhances accuracy, context-awareness, and efficiency.
- Industry-Specific Use Cases Insight into how RAG is revolutionizing sectors like banking, healthcare, and manufacturing.
- RAG’s Value in Addressing Business Challenges and Continuity Discussing how RAG supports critical business functions and decision-making processes.
What is Retrieval-Augmented Generation (RAG)?
Retrieval-Augmented Generation represents a paradigm shift in AI technology, bridging the gap between static knowledge and dynamic, real-time information retrieval. At its core, RAG integrates large language models (LLMs)—which are adept at understanding and generating human-like text—with external data sources that can be queried in real time. This integration allows AI systems to access and incorporate the most recent and relevant information when generating responses.
Traditional AI models operate based on a fixed dataset acquired during the training phase. While these models can be powerful, they are inherently limited by the scope and recency of their training data. This means they may lack awareness of events, data, or developments that occurred after their training cutoff, leading to outdated or incomplete responses.
RAG addresses these limitations by equipping AI models with the ability to retrieve information from external databases, APIs, or knowledge repositories at the moment of generating a response. For example, when a user poses a question, the AI model can perform a search across designated data sources, retrieve pertinent information, and then incorporate that information into its generated response. This results in answers that are not only linguistically coherent but also enriched with the latest data, ensuring that users receive accurate and timely information.
Moreover, RAG’s architecture allows the AI to understand the context of a query more deeply. By considering both the user’s intent and the most relevant data available, RAG systems can provide nuanced answers that are tailored to specific needs. This makes RAG particularly valuable in domains where information changes rapidly or where precise, context-specific answers are crucial.
How RAG Enhances AI Performance
The integration of retrieval mechanisms into AI models through RAG significantly amplifies their performance and utility. Here are some of the critical ways RAG enhances AI capabilities:
Improved Accuracy
One of the most significant benefits of RAG is the enhancement of response accuracy. By accessing up-to-date information, RAG-powered models reduce the risk of providing outdated or incorrect answers. This is especially important in fields where information changes frequently, such as news, regulations, or market data. For instance, a RAG system can provide the latest stock prices, breaking news events, or updated policy information, ensuring users receive the most current data.
Contextual Relevance
RAG systems excel at aligning responses with the user’s intent by leveraging real-time data retrieval. They can interpret the nuances of a query and fetch information that is directly relevant to the context. This results in answers that are not only accurate but also highly pertinent to the user’s needs. For example, in customer support, a RAG system can access a customer’s account information and provide personalized assistance.
Scalability
As enterprises grow and their data repositories expand, maintaining AI models that can handle vast amounts of information becomes challenging. RAG offers a scalable solution by decoupling the language generation component from the data storage. Instead of retraining models to include new data, RAG systems can dynamically access information as needed. This means that as new data becomes available, it can be immediately utilized without the need for time-consuming retraining processes.
Efficiency
RAG enhances efficiency by reducing the dependency on extensive training cycles. Traditional AI models require periodic retraining to incorporate new data, which can be resource-intensive and slow. With RAG, the AI model can retrieve the latest information in real time, minimizing downtime and ensuring that responses are always based on the most current data. This efficiency translates into faster deployment times and the ability to adapt quickly to changing information landscapes.
Industry Use Cases for RAG
The versatility of RAG makes it applicable across various industries where access to real-time, accurate information is paramount. Below are some industry-specific use cases illustrating how RAG is transforming operations:
Banking
In the financial sector, timely and precise information is critical. RAG supports customer service representatives and automated chatbots by providing instant access to the latest financial data, regulatory changes, and personalized account information.
Example Scenario: A customer inquires about the latest mortgage rates and how they might be affected by recent market changes. A RAG-powered AI assistant can retrieve the most current rates, consider the customer’s credit profile, and provide personalized advice, enhancing the customer experience and trust in the institution.
Healthcare
Healthcare professionals rely on the most recent medical research and patient data to make informed decisions. RAG enhances AI systems by enabling access to up-to-date medical journals, clinical trial results, and patient records in real time.
Example Scenario: A doctor seeks information on the latest treatment protocols for a rare disease. A RAG system can retrieve and summarize the most recent studies, offering valuable insights that support better patient outcomes.
Manufacturing
In the manufacturing industry, efficiency and precision are key. RAG streamlines operations by providing real-time data on production processes, supply chain logistics, and equipment maintenance schedules.
Example Scenario: A plant manager needs to optimize the production schedule due to a sudden change in demand. A RAG-enabled system can retrieve current inventory levels, supplier delivery times, and workforce availability to recommend the most efficient production plan.
Addressing Real-World Business Challenges with RAG
RAG is not just a technological advancement; it’s a strategic tool that addresses numerous business challenges and supports continuity in operations. Here’s how RAG contributes to various aspects of business management:
Enhanced Decision-Making
Access to accurate, real-time data empowers leaders to make informed decisions quickly. RAG systems can aggregate and analyze data from multiple sources, presenting actionable insights that drive strategic initiatives.
Regulatory Compliance
Staying compliant with ever-changing regulations is a significant challenge. RAG ensures that organizations have the most current regulatory information, reducing the risk of non-compliance penalties.
Customer Satisfaction
By providing accurate and timely responses, RAG enhances customer interactions. Whether through chatbots or support staff, the ability to address customer needs promptly fosters loyalty and trust.
Operational Efficiency
Streamlined access to data allows employees to focus on strategic tasks rather than spending time searching for information. RAG automates data retrieval, reducing operational bottlenecks.
Product Innovation
RAG facilitates innovation by analyzing market trends, customer feedback, and technological advancements. This information helps companies develop products that meet evolving customer needs.
Risk Management
Effective risk management relies on comprehensive data analysis. RAG provides the necessary information to assess potential risks accurately, enabling proactive mitigation strategies.
Personalized Marketing
Understanding customer preferences is key to successful marketing. RAG systems can analyze customer behavior and preferences, allowing for highly targeted and personalized marketing campaigns.
Supply Chain Optimization
RAG enhances supply chain management by providing real-time visibility into inventory levels, supplier performance, and logistics. This information enables companies to optimize supply chain operations, reduce costs, and improve delivery times.
Employee Training
Continuous learning is vital in today’s fast-paced business environment. RAG provides employees with up-to-date training materials and resources, supporting professional development and keeping skills sharp.
Crisis Management
During unexpected events or crises, timely information is crucial. RAG aggregates relevant data quickly, enabling organizations to respond effectively and minimize negative impacts.
Competitive Intelligence
Staying ahead of competitors requires insight into their activities and market trends. RAG systems can monitor and analyze competitor data, providing valuable intelligence that informs strategic decisions.
Sustainability Initiatives
As businesses focus more on sustainability, RAG provides access to the latest environmental data and best practices. This supports efforts to operate responsibly and meet sustainability goals.
Global Expansion
Entering new markets involves understanding local regulations, cultural nuances, and market conditions. RAG equips organizations with the necessary insights to navigate global expansion successfully.
Conclusion and Call to Action
The adoption of Retrieval-Augmented Generation represents a significant leap forward in AI capabilities for enterprises. By integrating real-time data retrieval with advanced language generation, RAG empowers organizations to operate with greater precision, agility, and responsiveness. The benefits of RAG extend across various facets of business, from enhancing customer experiences to driving innovation and maintaining a competitive edge.
For enterprises looking to stay ahead in a rapidly changing technological landscape, embracing RAG is not just an option—it’s a necessity. Investing in RAG positions your organization as a leader in AI, ready to tackle challenges with confidence and sophistication.
Next Steps:
- Explore RAG Training Courses: Gain a deeper understanding of how RAG can be integrated into your business strategies.
- Assess Your Data Infrastructure: Ensure that your data sources are accessible and compatible with RAG systems.
- Engage with AI Experts: Consult with professionals to tailor RAG solutions that meet your specific business needs.
- Pilot RAG Projects: Start small with pilot programs to test the effectiveness of RAG in your organization.
- Stay Informed: Keep abreast of the latest developments in RAG technology to continually enhance your AI capabilities.
By taking these steps, your organization can fully leverage the power of Retrieval-Augmented Generation, driving success and innovation in the digital age.